Predictive Iterative Learning Control
Combining Data-driven Control with Additive Manufacturing - My first exposure to AI and ML 🤖
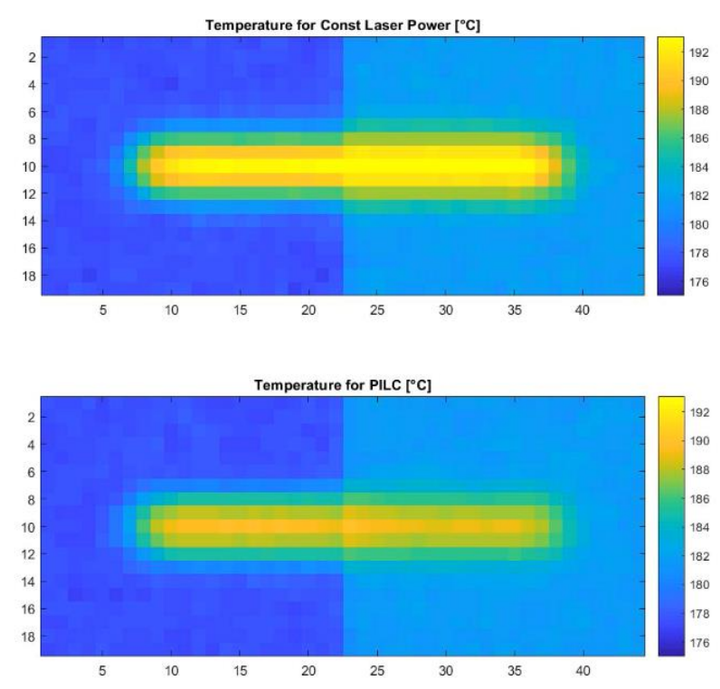
During my Master’s research at the University of Texas at Austin, I focused on enhancing the precision and quality of Selective Laser Sintering (SLS) through advanced data-driven control techniques. My work introduced a Predictive Iterative Learning Control (PILC) framework that optimizes the laser power profile dynamically, reducing deviations in post-sintering temperatures and improving overall part quality.
Challenges in Selective Laser Sintering
SLS, a subset of Powder Bed Fusion (PBF) additive manufacturing, builds parts layer by layer by fusing powder with a laser. The process is highly sensitive to temperature variations, which can introduce defects such as residual stresses, geometric inaccuracies, and suboptimal mechanical properties. Existing SLS machines primarily operate in an open-loop fashion, with static laser power parameters that fail to adapt to changing thermal conditions. Conventional feedback controllers, such as PID control, lack predictive capabilities and are inadequate for capturing the complex thermal dynamics of the process.
Development of the PILC Controller
To address these limitations, I developed a PILC controller that leverages machine learning-driven predictive modeling and iterative learning principles. The controller operates as follows:
Infrared (IR) Measurement Integration: At the beginning of each layer, the system captures pre-scan IR images to assess initial powder bed conditions.
Machine Learning-Based Black Box Model (BBM): The BBM predicts the maximum post-sintering temperature profile along the laser scan path based on historical machine data and the pre-scan IR image.
Optimization of Laser Power Profiles: The PILC controller iteratively refines the laser power profile using an error-based learning approach. If the predicted temperature deviates significantly from the desired value, an offline training cycle updates the power settings using:
Iterative Learning Control (ILC): A control strategy that refines the laser power based on past error patterns.
Machine Learning Models: Including deep neural networks, polynomial regression, and k-nearest neighbors (KNN), which enable accurate temperature prediction while maintaining computational efficiency.
Adaptive Online Learning: If subsequent layers exhibit similar conditions, the system refines laser power in real time using updated IR measurements, ensuring continuous optimization across layers.
Simulation and Performance Evaluation
To validate the effectiveness of the PILC controller, I conducted heat transfer simulations comparing PILC-controlled laser power profiles with constant power profiles. Key findings include:
Improved Temperature Uniformity: The PILC approach significantly reduced thermal inconsistencies across scanlines, maintaining a uniform post-sintering temperature.
Precision in Complex Geometries: The controller adapted effectively to complex part geometries, preventing localized overheating and warping.
Scalability and Learning Efficiency: The PILC framework improved progressively with each iteration, demonstrating convergence toward an optimal laser power profile with minimal computational overhead.
Future Work and Implications
My research establishes a robust foundation for integrating advanced control techniques into additive manufacturing. Future extensions of this work include:
Experimental Validation: Implementing the PILC controller in real-world SLS systems to assess its impact on mechanical properties and part integrity.
Multivariate Optimization: Expanding the control framework to optimize multiple process parameters simultaneously, such as laser speed and powder bed preheating.
Hybrid Modeling Approaches: Combining data-driven BBMs with physics-based Grey Box Models (GBMs) for enhanced predictive accuracy.
Conclusion
By bridging the gap between modern control theory and machine learning, my research provides a significant step forward in SLS process optimization. The PILC framework not only improves part quality and repeatability but also enables real-time adaptation to process variations, marking a critical advancement toward intelligent additive manufacturing systems.